Structured Streaming is a scalable and fault-tolerant stream processing engine built on the Spark SQL engine. inshort Structure streaming rebuild streaming functionalities on top of Catalyst and Data Frames.
Structured Streaming is the first API to build stream processing on top of SQL engine,in traditional streaming we process only real-time events, structured streaming is a novel approach of processing structured data of either a discrete table of data (where you know all the data) or an infinite table of data (where new data is continuously arriving). When viewed through this structured lens of data – as a discrete table or an infinite table.
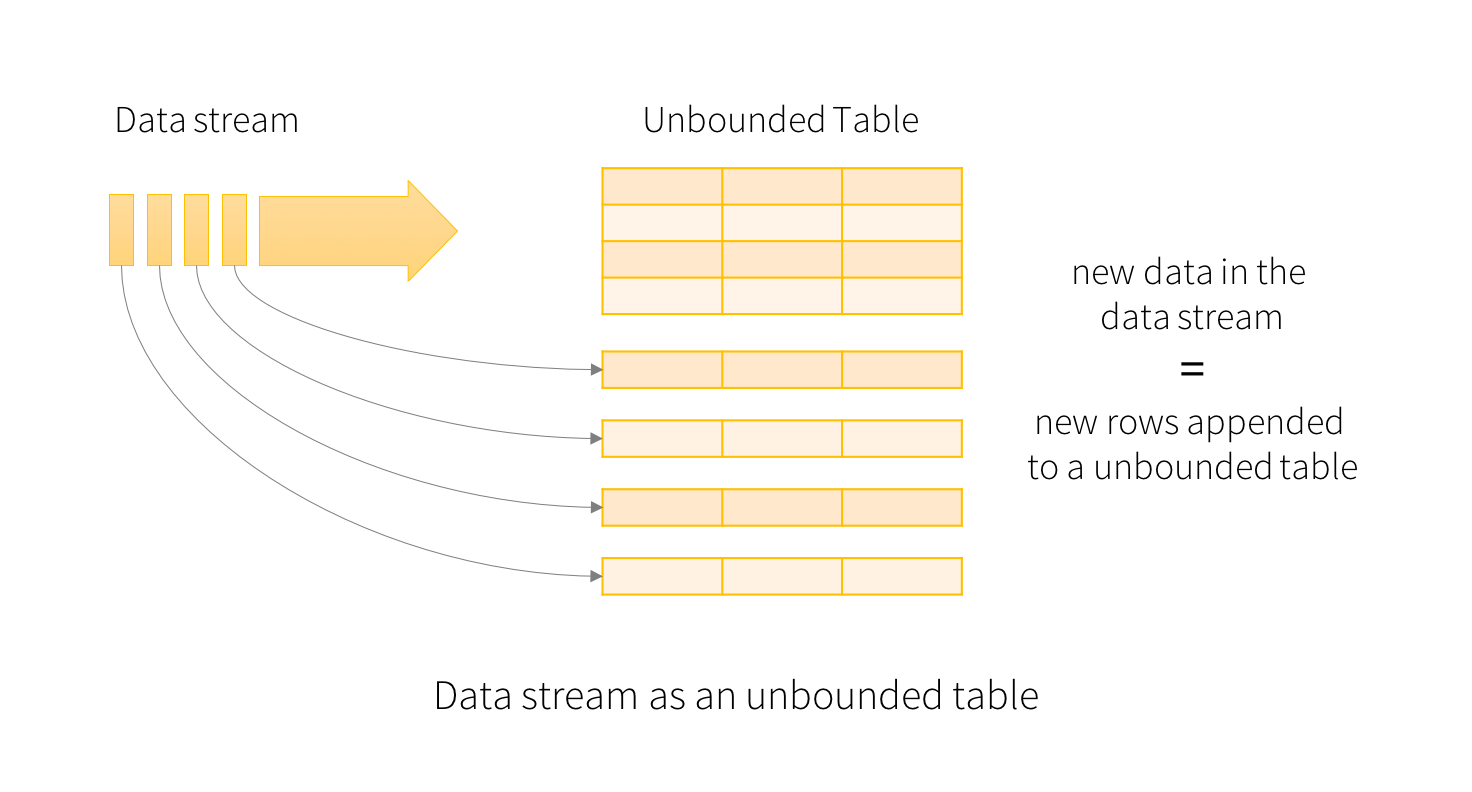
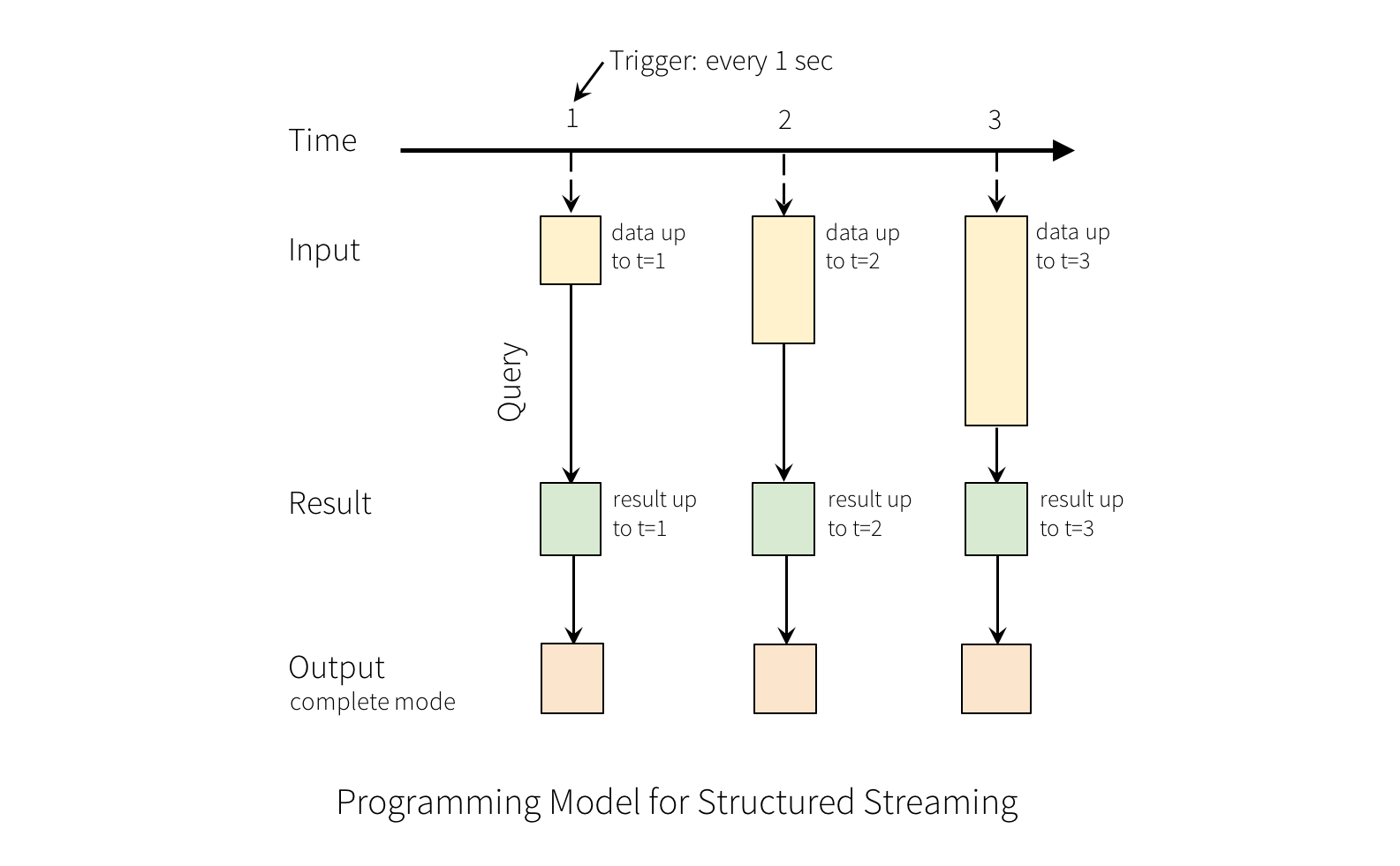
Logically, each input stream is an appendonly table (i.e. DataFrame), where records can arrive in any order across our system processing time (event time is just a field in each record).
Users define queries as just traditional SQL or DataFrame queries on this whole table,which return a new table whenever they are executed in processing time.
Users set a trigger to say when to run each query and output, which is based purely on processing time. The system makes best effort to meet such requirement. In some cases, the trigger can be gas soon as possibleh.
Finally, users set an output mode for each query. The different output modes are:
Delta : Although logically the output of each query is always a table, users can set a "delta" output mode that only writes the records from the query result changed from the last firing of the trigger.
- These are physical deltas and not logical deltas. That is to say, they specify what rows were added and removed, but not the logical difference for some row.
- Users must specify a primary key (can be composite) for the records. The output schema would include an extra "status" field to indicate whether this is an add, remove, or update delta record for the primary key.
Update( inplace ) : Update the result directly in place (e.g. update a MySQL table) ,similar to delta, a primary key must be specified.
Complete : For each run of the query, create a complete snapshot of the query result.
Repeated queries(RQ) :RQ simply states that the majority of streaming applications can be seen as asking the same question over and over again,the results from RQ are identical to running each query manually at various points in time, and saving either its whole output or just the difference.
Example : How many credit card transactions happen today
Select * from tbl_credit_card_transactions group by card_type
- https://spark.apache.org/docs/latest/structured-streaming-programming-guide.html
0 comments:
Post a Comment